Harnessing Production Machine Learning for Business Efficiency
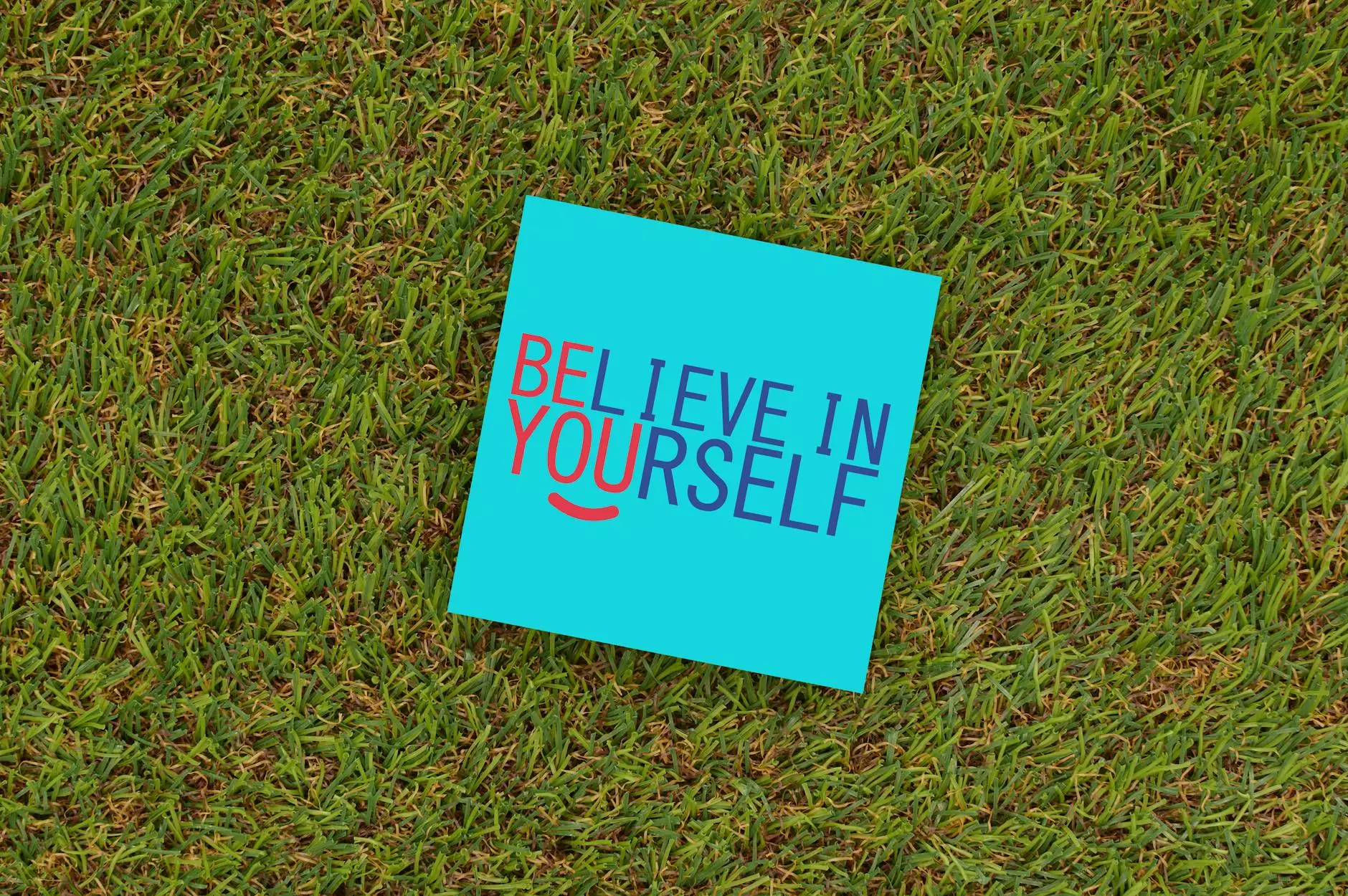
Production machine learning is not merely a buzzword in the realm of business technology; it is a powerful tool that can drive significant transformations across various sectors. This article delves deep into what production machine learning entails, its impacts on business process automation, content management services, and data governance systems, and how organizations can effectively implement these technologies for enhanced productivity and decision-making.
Understanding Production Machine Learning
Production machine learning involves the application of machine learning methods in production environments where models are continuously trained and updated based on real-time data. It is distinct from traditional machine learning, where models are often developed in isolated research settings without a direct connection to operational systems. The key aspects of production machine learning include:
- Real-Time Data Processing: The ability to process and analyze data as it is generated, allowing businesses to make timely decisions based on the latest information.
- Model Deployment: Techniques for integrating machine learning models into existing operational workflows, ensuring that they can be utilized effectively across departments.
- Continuous Learning: Mechanisms that allow machine learning models to adapt and improve over time as new data becomes available.
The Importance of Production Machine Learning in Business
In today's fast-paced business environment, the need for efficiency and agility cannot be overstated. Here are several ways production machine learning enhances business performance:
1. Automation of Business Processes
One of the most significant benefits of production machine learning is its capacity to automate complex business processes. By implementing machine learning algorithms, businesses can:
- Streamline Operations: Automation reduces manual tasks, leading to improved efficiency and reduced operational costs.
- Reduce Errors: Algorithms can analyze vast amounts of data consistently and accurately, mitigating the chances of human error.
- Enhance Decision-Making: Data-driven insights enable leaders to make informed decisions quickly.
2. Improved Data Governance Systems
Effective data governance is crucial for any organization seeking to leverage its data fully. Production machine learning can aid in:
- Data Quality Assurance: Machine learning models can automatically flag and correct anomalies in data sets, ensuring high-quality data is being used for analysis.
- Regulatory Compliance: Automated checks can be built to ensure compliance with industry regulations regarding data handling and management.
- Enhanced Security: Machine learning can contribute to stronger data protection measures by identifying and predicting security threats.
3. Content Management Services Reinvented
The integration of production machine learning into content management can deliver exceptional results. Businesses can:
- Personalize User Experience: By analyzing user data, businesses can tailor content to meet the specific needs and preferences of their audience.
- Optimize Content Delivery: Machine learning algorithms can determine the best times to distribute content, ensuring maximum engagement.
- Automate Content Creation: Natural language processing allows for the automated generation of reports, summaries, or even articles, freeing up valuable resources.
Implementing Production Machine Learning: Steps for Success
Integrating production machine learning into your business requires a strategic approach. Here are the steps to ensure successful implementation:
1. Define Business Objectives
Before diving into production machine learning, it is vital to have clear objectives. Determine what specific problems you want to solve and how machine learning will help achieve your goals.
2. Assess Current Infrastructure
Evaluate your existing data infrastructure. Consider whether your systems can handle the requirements for data collection, storage, and processing necessary for machine learning applications.
3. Data Collection and Preparation
Data is the foundation of machine learning. Gather quality data sets relevant to your business objectives and ensure they are cleaned and preprocessed for model training.
4. Choose the Right Algorithms and Models
Select the appropriate machine learning algorithms based on your objectives. Depending on the complexity of the tasks and available data, various models may be employed, including supervised, unsupervised, or reinforcement learning approaches.
5. Continuous Testing and Optimization
Once models are deployed, continuously test their performance. Collect feedback, monitor outcomes, and refine the models to ensure they meet business needs effectively.
Case Studies: Success Stories of Production Machine Learning
Understanding the practical applications of production machine learning can drive better insights into its benefits. Here are a few case studies that demonstrate its effectiveness:
1. E-commerce Personalization
An online retail giant implemented machine learning to analyze customer browsing patterns and purchasing behavior. As a result, the company could recommend products tailored to individual consumers, which increased conversion rates by over 20%.
2. Predictive Maintenance in Manufacturing
A major manufacturing firm integrated machine learning algorithms into their maintenance routines. By predicting equipment failures before they happened, the company streamlined operations, significantly reduced downtime, and saved millions in repair costs.
3. Enhanced Fraud Detection
A financial institution utilized production machine learning to refine its fraud detection system. With AI-driven analytics, the bank could assess transaction patterns in real time, which drastically improved its detection rates and reduced fraudulent losses by 30%.
Future Trends in Production Machine Learning
The landscape of production machine learning is rapidly evolving. Here are some anticipated trends that organizations should keep an eye on:
- Increased Automation: More processes will become automated as machine learning models evolve, resulting in even less human intervention in operational workflows.
- Integration with Edge Computing: The combination of machine learning with edge computing will allow for faster data processing at the source, enhancing responsiveness.
- Ethical AI Practices: As machine learning becomes more integrated into businesses, the focus on ethical AI development and usage will grow, ensuring transparency and fairness in automated decisions.
Conclusion: Embracing Production Machine Learning
In conclusion, production machine learning stands at the forefront of technological innovation in business. By embracing this powerful tool, organizations can not only enhance operational efficiency but also foster a culture of continuous improvement and innovation. As we move forward, the integration of production machine learning into your business processes will not just be advantageous; it may become essential for survival in an increasingly competitive landscape.
For organizations looking to implement these advancements, partnering with experienced service providers specializing in content management services, business process automation, and data governance systems can offer the insight and technical support necessary for navigating this complex journey.
By staying ahead of the curve with production machine learning, businesses can unlock unprecedented opportunities for growth and innovation, ensuring they remain leaders in their respective industries.