The Comprehensive Guide to Labelling Tool Machine Learning
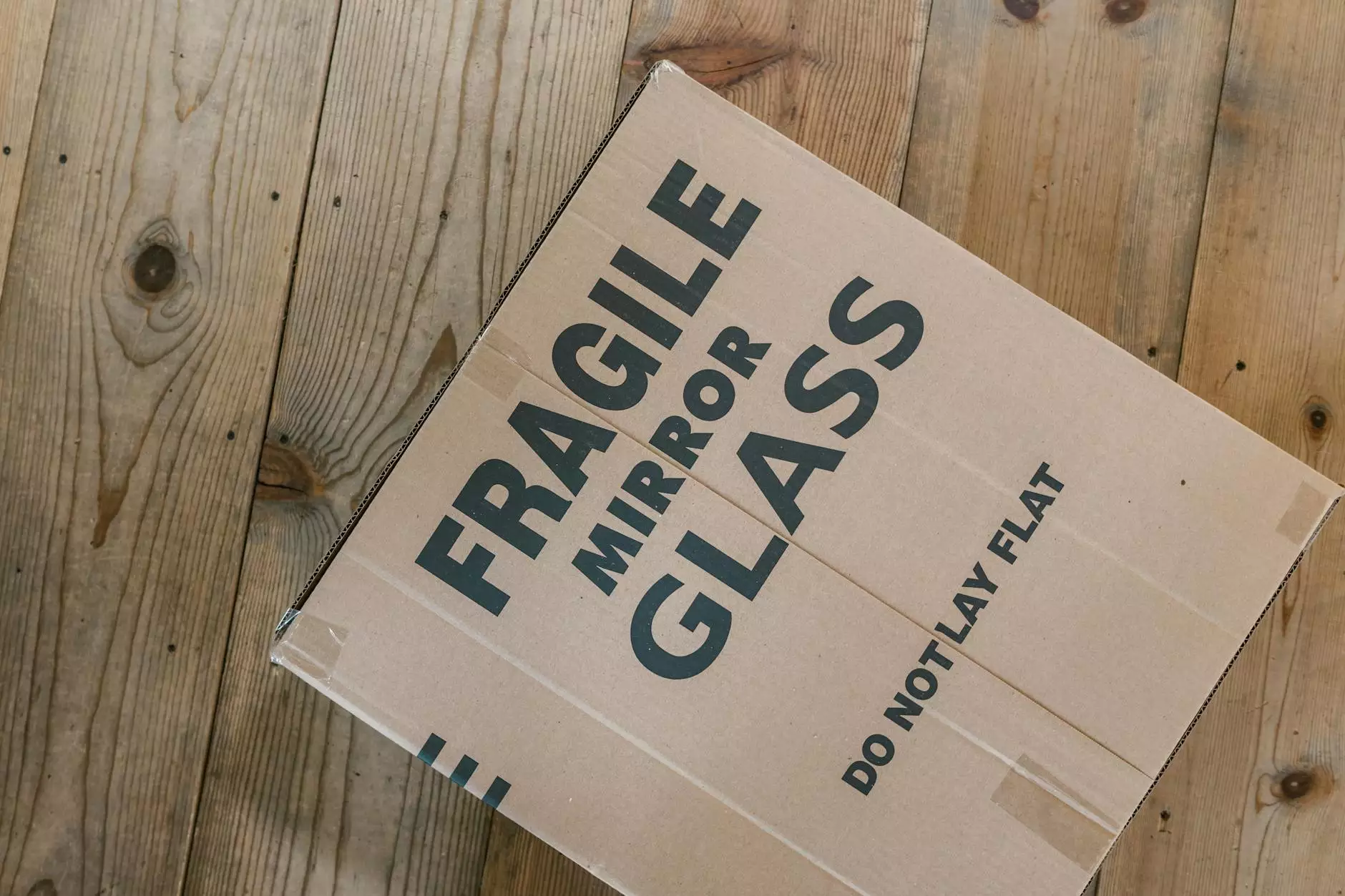
The dynamic world of machine learning is revolutionizing industries, and at the core of this transformation lies a crucial process: data annotation. Within this context, the labelling tool machine learning has emerged as a powerful solution that streamlines how companies handle vast amounts of data. In this article, we will delve deep into the significance of labelling tools in machine learning, their applications, advantages, and how they can elevate your business to new heights.
Understanding Data Annotation
Data annotation is the process of labeling or tagging data to make it understandable for machine learning algorithms. This process is essential for training models to recognize patterns, make predictions, and perform various tasks accurately. Here are some important aspects:
- Types of Data: Data can come in various forms, including text, images, audio, and video. Each type requires different annotation techniques.
- Machine Learning Algorithms: These algorithms learn from annotated data, allowing them to make informed decisions based on new, unseen data.
- The Importance of Quality: High-quality annotations are vital. Inaccurate labels can significantly diminish the performance of a model.
The Role of Labelling Tools in Machine Learning
As the volume of data continues to skyrocket, manual data annotation becomes increasingly impractical. This is where labelling tool machine learning steps in. These tools leverage automation and advanced algorithms to enhance the efficiency and accuracy of the annotation process. Here's how they contribute:
1. Automation of Annotation Tasks
Labelling tools can automate repetitive tasks, allowing data scientists and analysts to focus on higher-level tasks. With features such as:
- Batch Processing: Handle large datasets at once, drastically reducing the time required for manual labeling.
- Smart Suggestions: Tools can use machine learning to suggest labels based on previously annotated data, thereby increasing speed and consistency.
2. Enhanced Accuracy
With machine learning algorithms aiding in the annotation process, accuracy is significantly improved. Some features that enhance precision include:
- Real-time Error Checking: Automated systems can flag discrepancies as they occur.
- Feedback Loops: Continuous learning from user input ensures the tool improves over time, refining its accuracy.
3. Scalability
Businesses often face the challenge of scaling their data annotation efforts. Labelling tools provide the capability to handle increased data volume without a corresponding increase in manpower. This is particularly beneficial for:
- Startups: Companies can scale their operations without substantial upfront investment in human resources.
- Large Enterprises: Corporations can maintain productivity levels even when processing vast datasets.
Choosing the Right Labelling Tool
The market is rife with different labelling tools, each catering to specific needs and industries. To select the most suitable labelling tool machine learning for your needs, consider the following factors:
1. Industry-Specific Features
Some labelling tools are tailored for specific industries. For instance, tools designed for autonomous vehicles may incorporate features for annotating images and videos, while tools for healthcare might focus on annotating medical texts or radiographs.
2. User-Friendly Interface
The user interface should be intuitive, enabling teams to navigate easily and reduce the learning curve. A good interface should include:
- Drag-and-Drop Functionality: Easy data upload and annotation.
- Clear Tutorials: Guidance for new users to improve onboarding.
3. Integration Capabilities
Choose labelling tools that can integrate seamlessly with your existing workflow. This will ensure:
- Smooth Data Transfer: Automate the movement of data between systems.
- Compatibility with Analysis Tools: Ensure your annotated data can be easily analyzed and utilized.
Benefits of Using Labelling Tool Machine Learning
The implementation of a proficient labelling tool machine learning system offers numerous advantages, which can significantly impact your business. Here’s a closer look:
Increased Productivity
With the automation of data annotation, teams can produce annotations faster than manual processes would allow. This productivity boost translates directly into enhanced project timelines and quicker go-to-market strategies.
Cost-Effectiveness
Investing in a quality labelling tool can yield significant cost savings. By reducing the need for extensive manpower, businesses can allocate resources more efficiently toward other critical areas.
Consistent Quality of Annotations
A labelling tool can provide consistent training datasets, leading to higher reliability in machine learning models. Consistency in annotations ensures that the algorithms learn better, resulting in improved output and accuracy in predictions.
Challenges of Data Annotation
Despite its advantages, data annotation does come with challenges that need to be understood and managed:
1. Complexity of Tasks
Some data types require intricate labelling techniques that may be difficult for automated tools to handle. These complex tasks often necessitate human intervention.
2. Keeping Up with Changes
Industries evolve rapidly, and so do the needs for data annotation. Machine learning models need to be retrained with updated data regularly, which can be resource-intensive.
3. Data Privacy Concerns
Handling sensitive data requires rigorous data privacy and security measures to prevent breaches and ensure compliance with regulations like GDPR.
Future Trends in Labelling Tools and Machine Learning
The intersection of labelling tools and machine learning is continuously evolving. Here are some trends that are shaping the future:
1. Collaborative Annotation Platforms
As we move forward, platforms where multiple users can work on annotation tasks simultaneously are gaining popularity. This collaborative approach enhances efficiency and allows for diverse input.
2. Improved AI-Assisted Annotation
AI will continue to advance, enabling more sophisticated suggestions during the labelling process. These innovations will further reduce the manual effort required while improving accuracy.
3. Domain-Specific Solutions
As more industries recognize the value of machine learning, the demand for tailored labelling tools designed for specific niches will increase, ensuring that unique requirements are met effectively.
Conclusion: Embracing Labelling Tool Machine Learning for Business Success
In a world driven by data, the significance of labelling tool machine learning cannot be overstated. By investing in robust annotation solutions, businesses position themselves to leverage the power of machine learning effectively. Not only do these tools enhance productivity and accuracy, but they also provide a competitive edge that is crucial in today's fast-paced technological landscape.
As organizations continue to embrace data-driven strategies, the smart adoption of labelling tools will be key to unlocking the full potential of machine learning initiatives. For any business looking to thrive in the data age, prioritizing effective data annotation through advanced labelling tools is an investment that will pay dividends in the long run.
Take Action: Explore KeyLabs.ai
If you're interested in enhancing your data annotation process through cutting-edge technology, explore the offerings of KeyLabs.ai, a leader in Data Annotation Tools and Data Annotation Platform. Equip your team with the best tools to succeed in the evolving landscape of machine learning.