Leveraging **Image Datasets for Classification** in Business
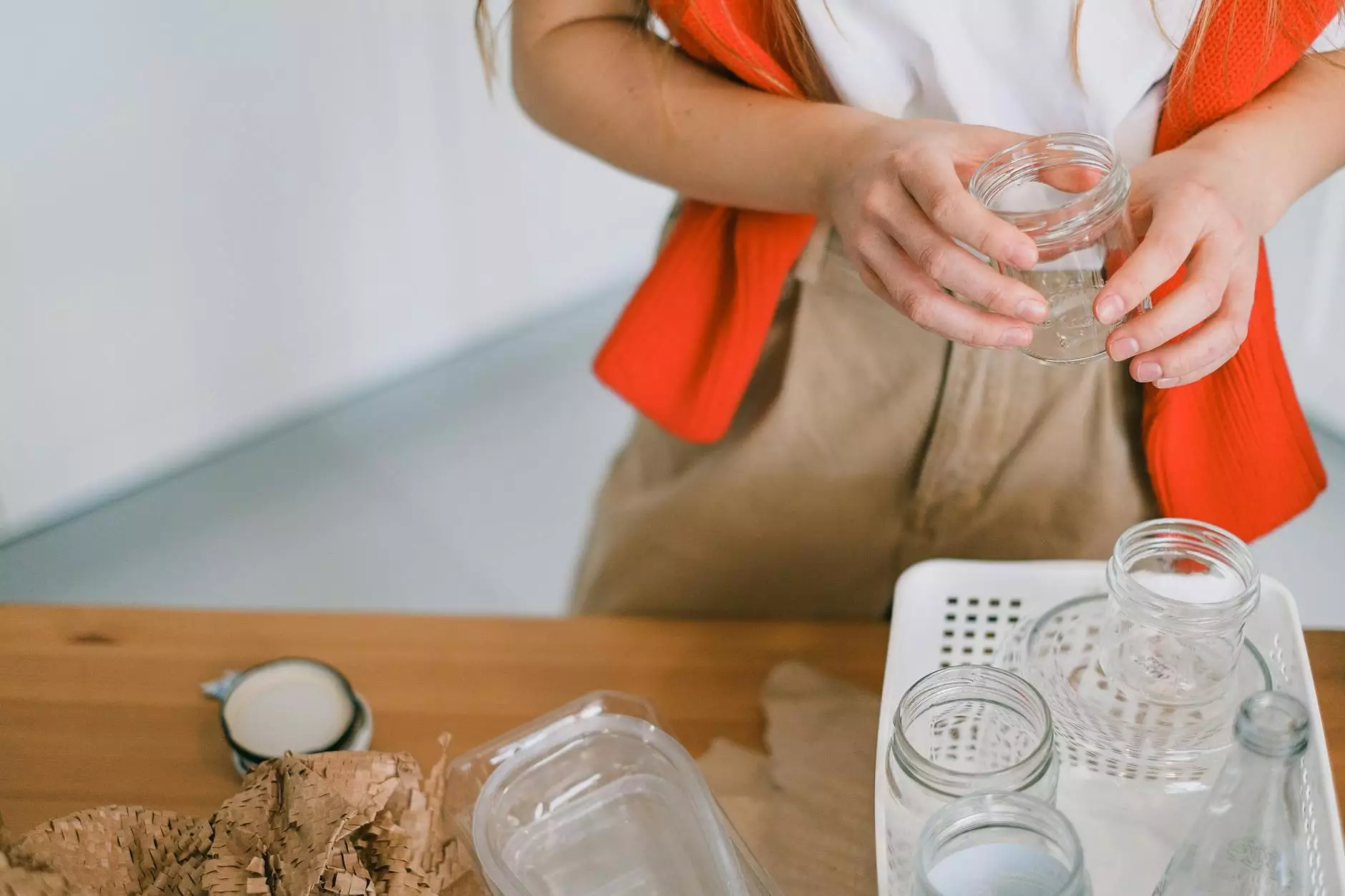
In today’s digital landscape, understanding the importance of image datasets for classification is pivotal for businesses aiming to harness the power of data. As organizations increasingly rely on advanced data analytics to drive decisions, the role of image classification becomes critical. Businesses that utilize effective data annotation tools and platforms like those offered by keylabs.ai are capitalizing on trends that amplify their operational efficiency and customer engagement.
What Are Image Datasets for Classification?
Image datasets for classification refer to collections of images that are categorized or labeled in a way that makes it easier for machines to understand and process them. These datasets serve as the foundation for training machine learning algorithms, particularly in the field of computer vision. In simple terms, they facilitate the teaching of a machine to recognize different types of images, enabling tasks such as:
- Object recognition: Identifying specific objects within images.
- Facial recognition: Distinguishing and validating human faces.
- Scene classification: Understanding different environments or settings in images.
- Medical image analysis: Interpreting images from scans for diagnostic purposes.
The Role of Data Annotation in Image Classification
Data annotation is the process of labeling datasets, and it’s essential for the performance of machine learning models. Through annotation, each image is marked with tags or boundaries, allowing algorithms to learn how to identify particular features. Here’s how data annotation bolsters image datasets for classification:
- Creates Standardized Data: Ensures consistency in labeling, which is crucial for training models effectively.
- Enhances Model Accuracy: High-quality, annotated data allows for better model performance, reducing errors in predictions.
- Facilitates Diverse Applications: Well-annotated datasets can be tailored for various sectors, including retail, healthcare, and security.
Types of Data Annotation Techniques
Various data annotation techniques can be applied to image datasets for classification, including:
Bounding Box Annotation
This technique involves drawing rectangles around objects in images. It’s particularly useful for tasks like object detection. For instance, a retail business might want to discern between products on shelves by bounding boxes around each item.
Semantic Segmentation
In contrast to bounding boxes, semantic segmentation assigns a label to every pixel in an image, offering a comprehensive understanding of what is present. This method is beneficial in more complex scenarios, such as autonomous driving where every detail matters.
Polygon Annotation
Polygon annotation allows annotators to draw free-form shapes around objects. This technique is particularly advantageous when dealing with irregularly shaped items, such as fruits or tools in industrial settings.
Why Businesses Need Image Datasets for Classification
Businesses across various industries are realizing the potential of utilizing image datasets for classification. Here’s why:
- Enhanced Customer Experiences: With image classification, businesses can better understand customer preferences and behaviors, tailoring services and marketing strategies accordingly.
- Streamlined Operations: Automated image classification can significantly reduce manual tasks, freeing up resources for more strategic initiatives.
- Informed Decision Making: Businesses can make data-driven decisions based on insights derived from image classifications, improving overall strategic approaches.
Implementing a Robust Data Annotation Strategy
To effectively leverage image datasets for classification, businesses need to implement a robust data annotation strategy. The following steps can guide organizations in this regard:
1. Define Objectives
Begin by clearly defining the objectives of the classification task. Understand what you hope to achieve—whether it's improving product recommendations or enhancing security measures.
2. Choose the Right Data Annotation Tool
Selecting an appropriate data annotation tool is essential. Tools offered by platforms like keylabs.ai ensure efficiency and ease of use, catering to various data annotation needs.
3. Train Annotators
Invest in training your annotators to ensure high-quality annotations. Annotators must understand the nuances of the classification task at hand to produce reliable datasets.
4. Quality Assurance
Establish a QA process that regularly reviews the quality of annotations. This step is critical to maintain the integrity of your data, allowing for optimal training of models.
5. Keep Iterating
Machine learning is an iterative process. Continually refine your datasets based on the performance of your models and feedback from their predictions.
Real-World Applications of Image Classification
The applications of image classification are vast and varied. Consider these notable examples:
Healthcare
In the healthcare sector, image datasets for classification are instrumental in diagnosing medical conditions from imaging scans, such as MRI or CT scans. Advanced algorithms can identify abnormalities faster and with higher accuracy than traditional methods.
Retail
Retail businesses utilize image classification to enhance product visibility on e-commerce platforms. By tagging images with relevant keywords, retailers can improve search engine visibility and ultimately boost sales.
Security
Security solutions rely heavily on image classification for facial recognition in public spaces or secure areas. Properly annotated datasets allow these systems to operate with high levels of accuracy, reducing false positives and streamlining surveillance processes.
Challenges in Data Annotation and Classification
While the advantages of utilizing image datasets for classification are numerous, there are challenges that organizations must navigate:
- High Cost of Annotation: Annotating images can be resource-intensive, requiring both time and financial investment.
- Maintaining Quality: Ensuring high-quality annotations can be challenging, particularly when dealing with large datasets.
- Dataset Bias: If the dataset is not diverse, it can lead to biased results, affecting the model's performance.
Future Trends in Image Classification
As technology advances, the future of image classification is bright. Some trends to keep an eye on include:
- Automated Data Annotation: Leveraging AI to assist in or automate the data annotation process, thus reducing time and costs.
- Deep Learning Models: Continued advancements in deep learning techniques that allow for more sophisticated image classification.
- Interdisciplinary Applications: Expanding the use of image classification beyond traditional fields into new domains, such as environmental monitoring and agriculture.
Conclusion
Embracing image datasets for classification empowers businesses to unlock insights from their data that were previously inaccessible. By investing in quality data annotation tools and platforms, like those from keylabs.ai, organizations can enhance their operational efficiencies, improve customer engagements, and drive innovation. As the realm of image classification continues to evolve, those who adapt and integrate these technologies will undoubtedly lead their industries into the future.