Unleashing the Power of Machine Learning Labeling Services
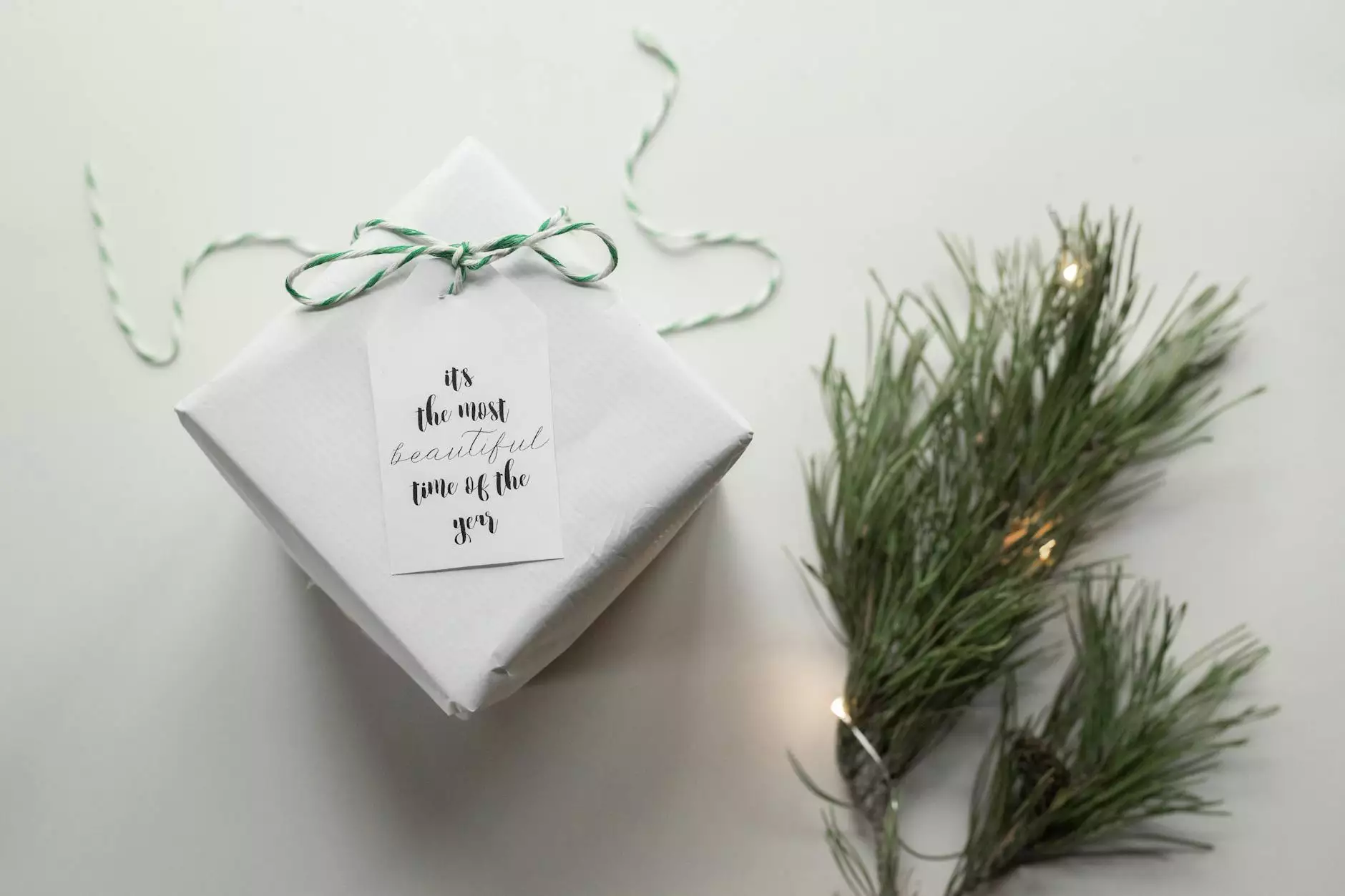
In recent years, the evolution of artificial intelligence (AI) has transformed industries and redefined the way we interpret and interact with vast amounts of data. At the heart of this transformation lies the critical process of data annotation, particularly through machine learning labeling services. This comprehensive article will delve deep into the significance of these services, how they operate, and the unparalleled advantages they offer businesses aiming to harness the full potential of AI technologies.
Understanding the Essentials of Data Annotation
Data annotation refers to the process of labeling and tagging data to make it comprehensible for machine learning algorithms. Without precise and accurate annotation, AI models may misinterpret data, leading to poor performance and flawed outcomes. Here are a few types of data that commonly require annotation:
- Image Annotation: This involves marking objects within images, enabling computer vision applications to recognize patterns and identify elements within visual data.
- Text Annotation: Textual data is enriched with tags indicating sentiment, entities, and intents, vital for natural language processing tasks.
- Video Annotation: This includes tracking objects throughout video sequences, beneficial for various applications ranging from autonomous vehicles to surveillance systems.
- Audio Annotation: Labeling audio clips for identifying speech patterns, language, and background noise enhances voice recognition systems.
The Integral Role of Machine Learning Labeling Services
As organizations increasingly rely on AI-driven solutions, the demand for machine learning labeling services has soared. These services are crucial for several reasons:
1. Enhancing Model Accuracy
High-quality labeled data is indispensable for machine learning models. Well-annotated datasets improve the precision of AI predictions, which translates directly into tangible benefits for businesses. Companies like Key Labs specialize in delivering meticulous data annotation that fuels model accuracy.
2. Leveraging Human Expertise
While automated systems can assist in data labeling, human annotators provide nuanced understanding and context. This expertise ensures that the machine learning labeling service generates data that aligns with real-world scenarios, fostering more reliable AI outcomes.
3. Saving Time and Resources
Outsourcing annotation tasks to specialized services allows companies to focus on their core business functions. The expertise required to label data efficiently fosters productivity, enabling internal teams to develop AI solutions without getting bogged down by the arduous process of data labeling.
Exploring the Advantages of Using Key Labs' Data Annotation Platform
Key Labs, recognized for its cutting-edge data annotation tools, offers a data annotation platform that streamlines the process of labeling large datasets. Below are notable advantages of utilizing their services:
1. Scalable Solutions
Whether a business deals with thousands or millions of data points, Key Labs' platform is designed to scale. Their robust infrastructure accommodates high volumes of data efficiently, ensuring timely delivery without compromising quality.
2. Multi-Domain Expertise
Key Labs brings together a diverse team of domain experts who can annotate data across various industries. Whether it's healthcare, finance, retail, or automotive, their extensive experience ensures that the data is relevant and applicable to the target domain.
3. Quality Assurance Measures
The quality of labeled data can make or break AI outcomes. Key Labs implements stringent quality control processes, whereinannotated data undergoes multiple verification stages to uphold accuracy and consistency. This dedication to quality sets them apart in the competitive landscape of machine learning labeling services.
Machine Learning Labeling Service in Action
Let’s explore some real-world applications of machine learning labeling services across various sectors:
1. Healthcare
In healthcare, annotated data is pivotal in developing AI models that assist in diagnosing diseases. For instance, annotating medical images such as X-rays or MRIs enables machine learning models to detect anomalies and assist doctors in making informed decisions.
2. Autonomous Vehicles
Self-driving technology heavily relies on well-annotated datasets. Providing clear labels for objects on the road, like pedestrians, traffic signs, and other vehicles, empowers AI systems to navigate safely and efficiently.
3. Retail and E-Commerce
Through text annotation of customer reviews and sentiments, retailers gain insights into consumer behavior. Understanding preferences and trends can significantly enhance marketing strategies and customer engagement.
Best Practices for Implementing Machine Learning Labeling Services
For organizations aiming to implement machine learning labeling services, adhering to best practices is crucial for optimizing results:
- Define Clear Objectives: Before initiating the annotation process, clearly outline the objectives of the machine learning model. What questions does it need to answer? This clarity guides the labeling process effectively.
- Choose the Right Tools: Leverage platforms like Key Labs that offer modern data annotation tools designed to enhance efficiency and accuracy. A well-equipped platform simplifies the annotation workflow.
- Ensure Continuous Feedback: Establish mechanisms for reviewing annotated data. Continuous feedback loops allow for the quick correction of errors, ensuring high-quality outcomes.
- Invest in Training: If using internal resources for annotation, invest in training programs that enhance the skills of annotators. Skilled annotators are vital for creating high-quality labeled datasets.
The Future of Machine Learning Labeling Services
As advancements in AI technology continue to evolve, so will the landscape of machine learning labeling services. Emerging trends to watch for include:
1. Automation and AI-Assisted Labeling
With the introduction of sophisticated algorithms, automated labeling processes will become more prevalent. AI can assist human annotators by suggesting labels or completing simple tasks, increasing overall efficiency.
2. Focus on Quality Over Quantity
The emphasis will gradually shift towards ensuring the quality of labeled datasets rather than merely increasing quantity. High-quality data is essential for fine-tuning AI models, and stakeholders will prioritize partners who excel in delivering precision.
3. Real-Time Data Annotation
With the rise of real-time applications, such as live video feeds and instant data analysis, the demand for real-time annotation services will escalate. Companies will seek solutions that can provide immediate and accurate labeling to facilitate instantaneous decision-making.
Conclusion
In summary, the role of machine learning labeling services in the data annotation landscape is irrefutable. As businesses endeavor to integrate AI into their operations, the effectiveness of their AI systems hinges on the quality and accuracy of the labeled data they utilize. By partnering with reliable providers like Key Labs that prioritize precision, scalability, and quality, organizations can unlock the full potential of their AI initiatives. Embrace the future today by prioritizing machine learning labeling services that empower your business to thrive in the data-driven era.